Yesterday I found this study about how it might be possible to optimize against LLM-based search engines (think Google Bard, Google SGE, Bing, ChatGPT, etc), AI Search or Generative Engines as the study calls them.
They coin this new approach: Generative Engine Optimization (GEO).
The research, conducted by teams from Princeton University, Georgia Tech, Allen Institute for AI, and IIT Delhi, evaluates which strategies that are effective in order to improve visibility in these types of search engines.
And this is interesting, as traditional SEO methods may not yield the same results with these new-age generative engines, which provide direct, comprehensive responses and could potentially decrease organic traffic to websites.
Therefore, SEO professionals must understand and adapt to this new paradigm as we will see more and more of this type of AI in Google search engine in 2024 and beyond.
By leveraging Generative Engine Optimization (GEO) methods, such as including citations, quotations from relevant sources, and statistics, SEOs can significantly boost a website's visibility in AI search results according to the study.
I previously discussed this a bit when Google Bard was introduced, explaining how its optimization could resemble strategies used for enhancing featured snippets.
The main findings of the study were:
- Focus on Impressions Metrics: Traditional metrics used in search engine optimization (SEO) are no longer sufficient for generative engines. Instead, GEO proposes a set of impression metrics that measure the visibility of citations and their relevance to the user query.
- Include including citations and quotations: The study evaluates various GEO methods and their effectiveness in improving source visibility. Notably, methods such as including citations, quotations from relevant sources, and statistics significantly boost source visibility by up to 40% in generative engine responses.
- Domain-Specific Optimization: The study demonstrates the importance of domain-specific optimization strategies. Different GEO methods perform better in certain domains, highlighting the need for targeted adjustments to enhance visibility. Like authoritative language worked best for improving historical content, citation optimization benefits factual queries, and statistics enhance law and government topics.
If you want the more meaty details, I have tried to outline them in the following.
What is AI Search or generative engines and why does it matter?
AI Search or Large Language Models (LLMs) represent the next generation of search engine technology.
These advanced systems, such as BingChat, Google's SGE, Perplexity or to degree ChatGPT, merge the capabilities of traditional search engines with the adaptability of generative models.
These new types of search engine, known as generative engines (GE), go beyond simply searching for information.
They generate multi-modal responses by synthesizing information from multiple sources.
Generative engines work by retrieving relevant documents from a database, such as the internet, and using large neural models to generate a response.
This response is grounded in the sources, ensuring attribution and providing a way for the user to verify the information.
While these engines offer significant advantages to both developers and users, they also pose challenges for website and content creators.
Unlike traditional search engines, generative engines can provide direct, comprehensive responses, which could lead to a decrease in organic traffic to websites and impact their visibility.
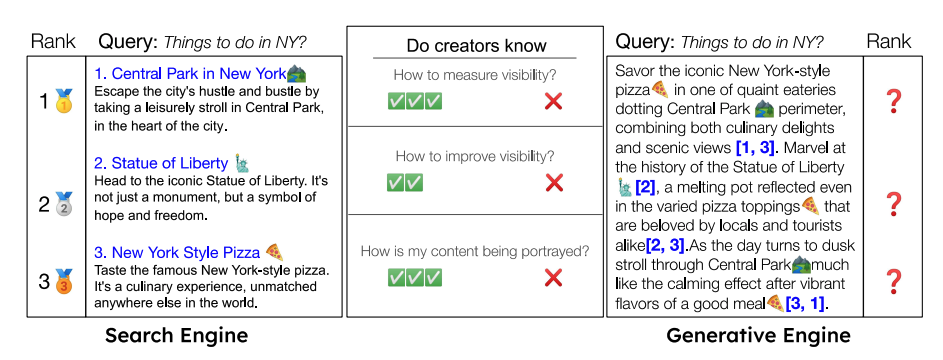
But, again Google have done this for quite some time with their more and more frequent featured snippet.
But of course GE or SGE takes this to a whole new level.
And as these engines continue to evolve, they will undoubtedly play a pivotal role in shaping the future of search technology.
The study: “GEO: Generative Engine Optimization”
In the study simply named “GEO: Generative Engine Optimization”, the researchers conducted experiments to evaluate the effectiveness of different Generative Engine Optimization (GEO) methods.
They used a benchmark called GEO-BENCH, which consisted of 10,000 diverse queries from different sources and domains.
For each query in the benchmark, the researchers randomly selected a source website and applied one of the GEO methods separately to optimize the content of that source. They generated multiple answers per query to ensure statistical reliability.
The performance of the GEO methods was evaluated using two metrics: Position-Adjusted Word Count and Subjective Impression.
The Position-Adjusted Word Count metric considered the word count and position of the citation in the generative engine's response.
The Subjective Impression metric incorporated multiple subjective factors to compute an overall impression score.
The relative improvement in impression for each source was calculated by comparing the impression scores of the optimized response to the baseline response without any optimization.
Additionally, the researchers analyzed the performance of the GEO methods across different categories and domains.
They identified the top-performing categories for each method, indicating the specific contexts in which each method was most effective.
The study used the Perplexity.ai search engine and an AI search engine modeled on Bing Chat. The researchers found that the results were similar across both platforms.

They evaluated the GEO methods on a subset of 200 samples from the test set to assess their performance in a real-world generative engine scenario.
The nine different optimization techniques evaluated
The researchers evaluated nine different GEO methods to optimize website content for generative engines.
To me it seems like these methods is a mix of classic SEO techniques (think keyword-usage, E-E-A-T, semantic richness, external links etc)
These 9 methods were:
- Authoritative: Modifies the text style of the source content to be more persuasive and authoritative, making claims with confidence.
- Keyword Stuffing: Modifies content to include more keywords from the user query, similar to traditional SEO optimization strategies.
- Statistics Addition: Modifies content to include quantitative statistics instead of qualitative discussion wherever possible, adding data-driven evidence.
- Cite Sources: Adds relevant citations from credible sources to support claims and provide attribution throughout the website content.
- Quotation Addition: Incorporates quotations from relevant sources to enhance the authenticity and depth of the website content.
- Easy-to-Understand: Simplifies the language and structure of the website content, making it more accessible and appealing to the generative engine and users.
- Fluency Optimization: Improves the fluency and readability of the website text, ensuring a smooth and coherent reading experience.
- Unique Words: Adds unique and intriguing vocabulary to the website content, making it stand out and increasing its appeal.
- Technical Terms: Incorporates technical terms and jargon relevant to the domain or industry, demonstrating expertise and catering to specific audiences.
.png)
The most effective GEO methods
They found that some methods were more effective in certain domains, while three strategies proved successful across all types of sites.
These top three strategies were Cite Sources, Quotation Addition, and Statistics Addition. These methods, requiring minimal changes to the actual content, improved the website's visibility by 30-40% compared to the baselines.
Interestingly, the researchers found that the effectiveness of optimization strategies varied depending on the knowledge domain.
For instance, the "Authoritative" optimization, which uses more persuasive language, worked best for content related to the Historical domain.
Meanwhile, the Citation optimization was most effective for factual search queries, and adding statistics proved beneficial for Law and government-related questions.

The research also revealed that some strategies were less effective than anticipated.
Using persuasive and authoritative tones in the content did not generally improve rankings in AI search engines.
Similarly, adding more keywords from the search query into the content (what we in classic SEO know as keyword stuffing if overdone), was not effective and performed worse than the baseline by 10%.
Are we to trust the study? I am not so sure
The researchers state that websites that are traditionally lower-ranked in SERP could significantly improve their visibility using GEO methods.
For instance, that the Cite Sources method led to a substantial 115.1% increase in visibility for websites ranked fifth in SERP.
This to me seems a bit random or too massive a change, which leads me to suspect that their methods are not 100% bulletproof.
Also they label “lower-ranked websites” as someone in fifth place in the SERPs. And that “many of these lower-ranked websites are often created by small content creators or independent businesses”.
To me, this misrepresents a misunderstanding when it comes to SEO. Lower-ranked websites are out of the top 10 for most queries, as today it requires a lot just to get to the first page. And most often first pages do not have small content creators in the first page.
Still, I find their study interesting, and I hope we see more of this as we move into the new era of GE or SGE.
While the study suggests that Generative Engine Optimization (GEO) could level the playing field for small content creators and independent businesses, there's a contrasting viewpoint that AI search or generative engines might instead favor larger, more credible websites.
This could potentially widen the gap between these entities and smaller businesses in the digital space.
Want to try the #1 AI Writer for SEO Copywriting?
Create anything from blog posts to product descriptions with 1-click AI drafts or our chat assistant. Powered by a next-gen SEO engine that ensures your content actually ranks. Try it now with a free trial→